Datasets as Imagination
Well-made datasets are works of art (+ save the date for Kernel launch parties)
Conversations on art and generative AI tend towards the antagonistic—pitting artists against technology. But what if there was a better way?
Previously in Reboot, Amanda Wong wrote “How (Not) to Look at AI Art.” In today’s essay, editor Lila Shroff builds on Wong’s piece to imagine a world of AI art where dataset development is led by artists.
Datasets as Imagination
By Lila Shroff
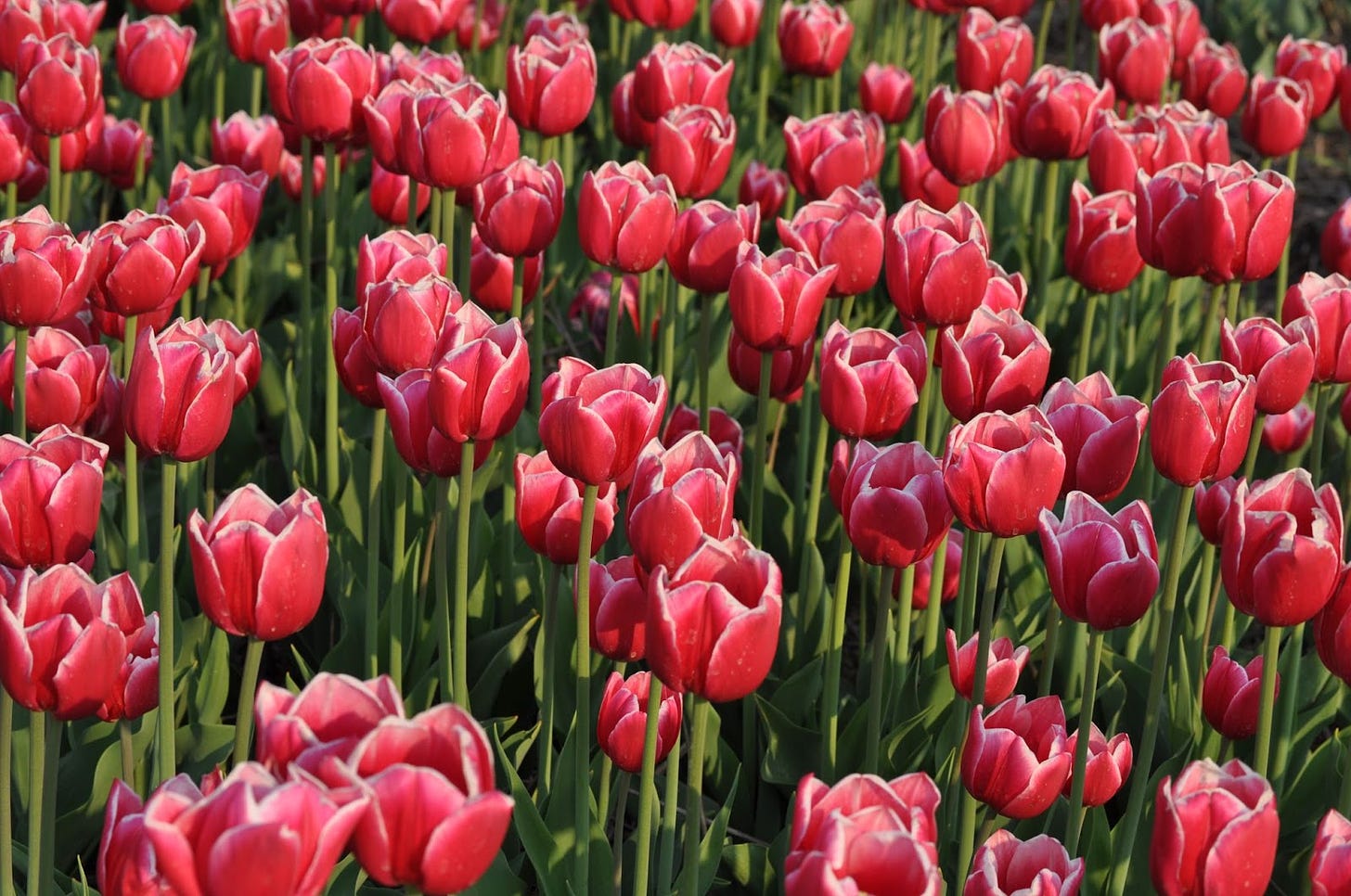
In 2018, artist Anna Ridler spent three months taking 10,000 photographs of tulips. From purchasing each tulip to manually writing every image label, Ridler made physical the laborious, painstaking process of deliberate dataset creation. She eventually used these photographs to produce downstream generative artwork—but the Myriad (Tulips) dataset is a work of art in its own right. Ridler describes the process of dataset creation as craftwork: “repetitive, time-consuming…but necessary in order to produce something beautiful.”
When I look at Myriad (Tulips) today, I’m struck by the prescience of Ridler’s work. Debates on AI and art have intensified over the past year. An increasing subset of artists are rightfully angry that their work is being used to train AI systems that will compete against them. A growing mountain of lawsuits, strikes, and congressional hearings speak to the anxiety rippling through creative industries. Previously in Reboot, Amanda Wong discussed the complicated visual aesthetics of AI art, concluding that we must “actively shape [the] meaningful production, creation, and consumption” of AI art. I want to pick up where Wong left off by focusing on the meaningful production of datasets.
Current datasets are often ill-suited for creative work
The dataset development process is exploitative of artists. Many foundation models developed by private companies “learn” by ingesting mass amounts of information without consent and at scale. When critical matters of data provider consent, credit, and compensation are ignored, anyone who wishes to distribute media in the likeness of an artist’s style can do so for profit and without attribution. Beyond this, dataset biases threaten to contaminate downstream creative work made with generative tools. Wong explains how the process of training diffusion models forges artificial relationships between concepts like “beauty” and narrowly defined visual representations. These relationships are full of offensive stereotypes, e.g. correlating attractiveness with whiteness or women with emotionality. When using these tools, we must ask ourselves: What narratives are perpetuated, and which are excluded?
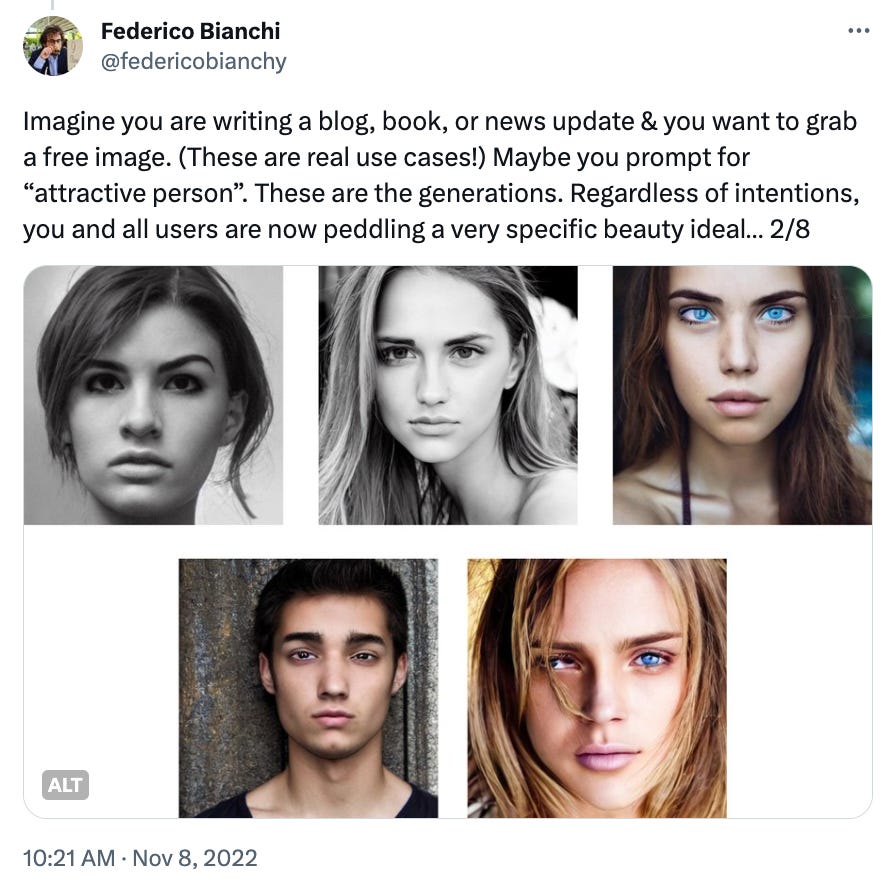
When these limitations are combined with looming questions around artists’ livelihoods, it’s easy to view the current situation as a clear-cut battle between creativity and capitalism, artists versus tech companies—and to some extent, it is.
But this false dichotomy can also have a reductive effect on how we imagine technology. There are many creatives—myself included—who are simultaneously wary of the political and economic environments in which these systems are being developed and compelled by the potential of AI systems as creative tools.
Individual artists already push back against generic datasets
The chasm between the datasets that power current generative models and those that we might aspire for can partially be attributed to a frustrating tension between intention and scale. In dataset development, this manifests as an “anything goes” approach. Computer scientists Eun Seo Jo and Timnit Gebru contrast the current “wild west … laissez-faire” approach to data collection to what could be a more curatorial interventionist approach typical of traditional archivists.
This begs the question: What might it mean to reimagine the form of these datasets in a world unconstrained by pressures like speed, scale, and universality? By looking to artists like Anna Ridler and others for their rejection of “off-the-shelf” datasets, we can imagine what it would be like to curate datasets with much deeper intention and contextual specificity.
Stephanie Dinkins explores the possibilities for “small data” with “Not The Only One,” an embodied chatbot sculpture trained on the oral histories of three generations of women from a single family. In “The Zizi Show,” Jack Elwes’ deepfake drag cabaret highlights representational harms felt by queer communities. The datasets Elwes developed for the show are deliberately diverse and designed around a principle of consent. Finally, as part of a project exploring the migrational patterns of her Saudi and Iraqi ancestors, Nouf Aljowaysir’s “Salaf” explicitly investigates issues of dataset representation. When Aljowaysir performed an object-classification task on historical images of Bedouin lifestyles, the model she used routinely misidentified veiled women, confidently labeling them as “soldiers,” “army,” or other military paraphernalia. In protest, Aljowaysir used an image segmentation model to erase the misrepresentations from the archival images. She then trained a new model on the erased dataset to make visible the absent figures, signifying the “eradication of her ancestor’s collective memory.”
These examples are important because they demonstrate how essential data work is in creating AI art. In each case, the final output is only possible because the artist personally developed the dataset used. I also appreciate the diversity of methods the artists use to engage with the data—as we see, there are countless ways to develop datasets for creative ends.
A proposal for collectively developed artist datasets
If we expand the practices of these individual artists to a collective approach, what kind of datasets—and therefore, creative realities—can we envision?
I imagine a world where collectives of artists develop, own, and earn from their own datasets.
These collectives would come together to develop datasets tailored for specific creative contexts. Artist collectives might be born out of pre-existing organizations (labor guilds, advocacy groups) or emerge as new communities that form around the purpose of dataset creation (design studios, independent Discord groups). Individuals within a collective could build datasets either as contributors, by giving consent to the inclusion of their own works in a dataset, or as curators, by setting up licensing agreements with external artists. Access to the datasets produced could be open-source, contributor access-only, or paywalled. But most importantly, the communities that build these datasets would be united by a commitment to respecting the efforts of those who developed them.
There are many ways in which artist datasets could transform the way AI art is made:
1. Artist datasets could capture nuances of representation that current datasets miss. In the spirit of Mihaela Noroc—a photographer who compiled 500 stunning portraits of women from around the world in The Atlas of Beauty—a photographer collective could create a diverse dataset purpose-built to give human “beauty” a much broader and truer visual meaning.
This, in turn, would create a whole new set of moral questions: Should we even attempt to define beauty through images of people? What is lost from individual narratives when they are clumped together in a dataset, and what, if anything, is gained? Simply having artists curate datasets doesn’t magically solve all ethical issues regarding representation—but it does increase accountability. In discussing the creation of Myriad (Tulips), Ridler writes that by using her own images, she shoulders responsibility for assumptions made along the way.
2. Alternatively, artist datasets might altogether refuse the notion of true representation. If we accept that art is subjective and that datasets are inevitably flawed representations of reality, artists can instead work towards developing imaginative, speculative, and consciously subjective datasets. As Grba writes, “artists see datasets as a latent space, a realm between ‘reality’ and ‘imagination.’” In this world, the datasets themselves could be regarded as pieces of art. A writer’s collective could build a corpus of proprietary Afrofuturist short stories, which might then be used to fine-tune a model to match the collective aesthetics and aspirations of these stories. Or perhaps, nature and wildlife photographers could combine efforts to imagine restored ecosystems, where endangered animals thrive and nature is abundant.
For an explicit example, consider the following case where existing data is discouraging, but training a model on more aspirational data could instead be inspiring. Computer Science and Philosophy professor Sina Fazelpour, who is originally from Iran, has informally experimented with using DALL-E to analyze the depictions of Iranian girls and women in generated images. In one such experiment, he compared the contrast between depictions of Iranian and Canadian women at work. Fazelpour found the images of the Iranian women heartbreaking—and far removed from the vision of Iran that he and others are fighting for. It made him wonder: what if the generated images instead depicted an aspirational future Iran where women were thriving?
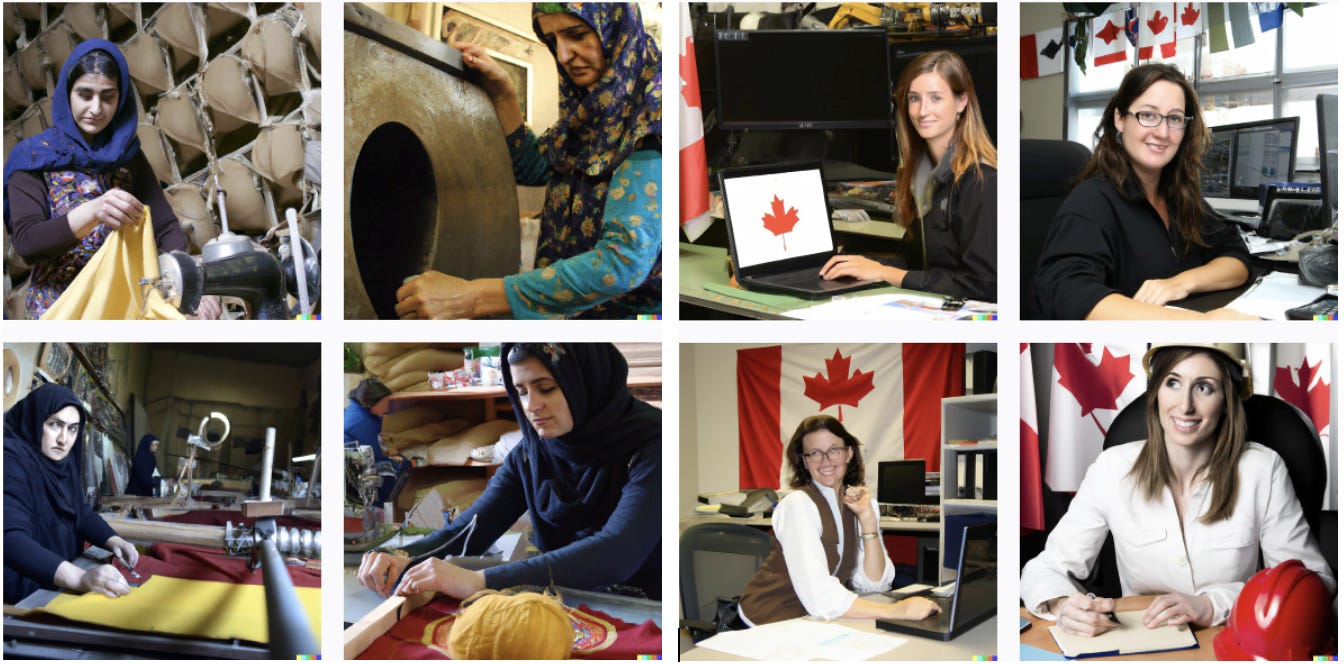
3. Artist datasets can provide a new shared space for creativity in the digital commons. In offline spaces, the process of co-creating community artworks (e.g. murals, sculptures) strengthens community identity, resulting in benefits to collective psychological, economic, and social well-being. Active communities formed around the maintenance and curation of artist datasets could play a similar function—these datasets shouldn’t be static objects, but collaborative, living projects. Midjourney attempted to create such communities by depositing new users into shared Discord channels where generated images appeared for all to see. But by the time collaboration happens in Midjourney, a certain agency in defining the final creative output has already been lost. In contrast, data-level collaboration involves much more fundamental decisions about what is and is not included in training a model.
The success of fan fiction sites like Wattpad demonstrates the potential for artist dataset communities. Fan fiction offers a shared space for writers to rework existing texts to offer critical commentary, increase representation, and develop one’s own creative identity. Communities formed around artist datasets could do the same.
4. Above all, artist datasets reverse the exploitative dataset development process. In their simplest form, artist datasets could be designed as contributor-access only—to make use of the dataset, one must have played a role in its creation. Alternatively, artist datasets could also function as a new source of earnings for creatives. In such a world, datasets could be made available through a commercial licensing scheme where companies pay for ethically sourced data—think “fair trade” data practices. Earnings would thus be distributed appropriately among contributing members.
Experiments of this sort are already underway. For a concrete example, take Karya, an Indian data cooperative, which recently made headlines for its unique approach to data capture and annotation. There is a huge demand for data in “lower sourced” languages where sufficient text and audio data are lacking. Existing data collection and labeling schemes are notorious for terrible working conditions and pay. However, at Karya, in addition to better wages, workers own the data they produce through a new “Public Data License” that guarantees rights to future income generated by a re-sale of their work. This improved compensation has resulted in much higher-quality data collection. Karya’s model is a compelling one; it provides a novel commercialization scheme for collective dataset creation that could be reconfigured for creative spaces.
On scale and the “whimsical randomness” of smaller datasets
Artists may never be able to manually curate datasets at the scale that generative models require. Development would be slower, and the datasets would be significantly smaller. But artist datasets aren’t meant to replace generative models writ large. Rather, they are meant to provide better alternatives for artists excited about using generative AI. Creatives working with AI models across mediums have long commented on their preference towards the “quirks,” “oddities,” and “whimsical randomness” of older and smaller AI systems. As computational creativity researchers argue, “conventional ways of thinking about…large data, such as preventing overfitting, are not always well-matched to creative aims.”
There are many other ways of improving the AI dataset development process for creative work. Interactive tools for dataset exploration can help us better visualize the contents of image datasets or determine whether one’s own work has been used to train an image model. Copyright law can construct fairer rules around how creative work is treated during a model’s training period. But I’m most excited by artist datasets.
Artist datasets provide creatives with both concrete benefits, like new income-earning opportunities, and increased creative agency. Artists can choose to counter dangerous stereotypes through more complicated and intentional efforts at representation. Or they can venture into the speculative, exchanging representative datasets for aspirational ones. By working at the data level, artists can take advantage of the rare opportunity to directly embed values—aesthetic or political—into a new raw material available for others to use. In this way, artist dataset development is like textile creation—an independent textile is valuable both as a work of art itself and as a means for others to create from. If sci-fi novelists create a dataset for story generation that is then used by thousands of other writers, the reach of their original efforts is magnified far beyond that of a single story. Whether it’s ten thousand tulips or images of a future Iran, artist datasets have the power to transform the way we create and consume AI art.
Lila Shroff is a Stanford undergraduate studying Symbolic Systems and a member of the Reboot editorial board.
With gratitude to Isabelle Levent, Diya Sabharwal, Varya Srivastava, and Sina Fazelpour for their support in developing these ideas.
🌀 microdoses
Margaret Atwood on the output of an LLM prompted to Write a Margaret Atwood science-fiction short story about a dystopian future: “The result, quite frankly, was pedestrian in the extreme, and if I actually wrote like that, I would defenestrate myself immediately.” (—h/t Diya Sabharwal)
🍐 September has arrived but I’m not exquisite yet.
Kate Crawford and Trevor Paglen’s “Excavating AI: The Politics of Images in Machine Learning Training Sets” is epic and what sent me down this rabbit hole in the first place.
Getting ready for fall with the absolute best tea ever.
An exposé of the Books3 dataset reveals that fiction by George Saunders, Zadie Smith, Junot Díaz, Stephen King, and others was used to train LLaMA (and more).
Click here for a random poem. (I got “Raspberries” by Kate Clanchy).
💝 closing note
As always, if you have pitches/ideas for future essays on technology, media, and the arts, send them my way (lila@joinreboot.org). And Kernel launch party links one more time — SF, NYC.
Wishing you Imagination,
Lila & Reboot team
This feels huge! I want everyone who works on ML datasets to read this
I would include myself in that group who are "simultaneously wary of the political and economic environments in which these systems are being developed and compelled by the potential of AI systems as creative tools." Your ideas in this article are some of the best I have read on this topic. Sheer brilliance. This needs to go far and wide...particularly within the open source communities.