How (Not) to Look at AI Art
When we have the power to ask for anything, what will we choose?
There is art, and there is content. We know AI can generate the latter. But what about the former? What makes something artistic? Beauty? Craft? Human intent? These are age-old questions, yet only superficially explored in most discussions about generative AI.
In today’s guest essay, AI engineer and art historian Amanda Wong offers a nuanced guide to modern looking—what might be lost (and found) in this new wave of creation.
P.S. Four more days to apply to join our editorial board! More at the bottom.
How (Not) to Look at AI Art
By Amanda Wong
In a recent visit to the Museum of Modern Art, I spent more time looking at people than at art. A sizable crowd was gathered in front of Refik Anadol’s “Unsupervised,” which vibrated with the rhythmic hum of a breathing machine. Anadol collaborated with the museum to train a machine learning algorithm on its collection to “interpret” and visually represent the intersections of objects, while also inventing new connections. As the colorful blobs crawl, surge, and fold into themselves like magma, “Unsupervised” looks as though it is about to break free from the wall and spill into the foyer.
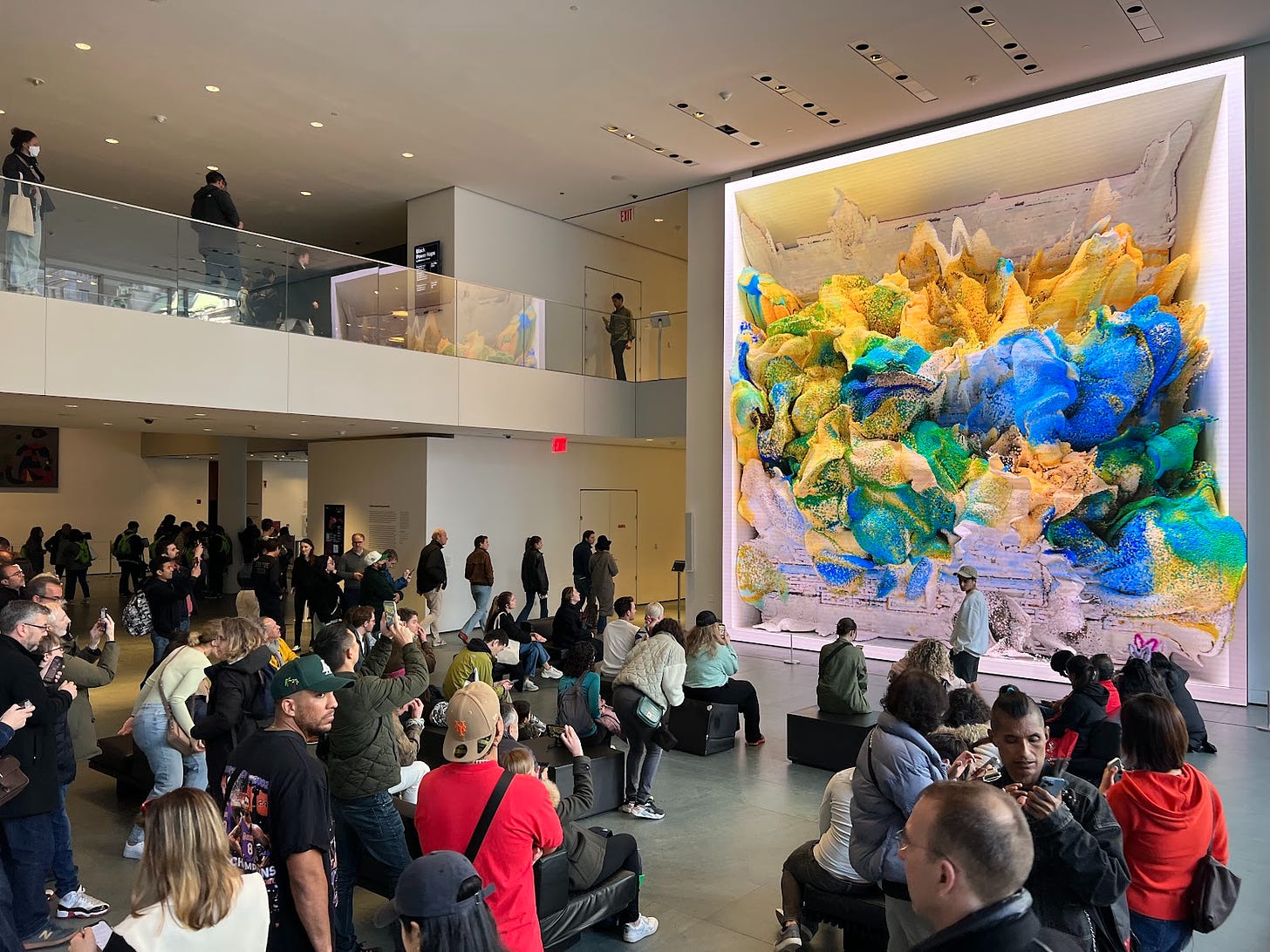
Its pixels danced in my periphery as I surveyed the audience. A couple pointed out shapes they saw among the pulsating forms, as though gazing up at clouds. A man said to his teenage son as they rushed past me, their eyes fixed on the work, “Isn’t it incredible? I don’t know what it is, but it’s amazing.”
“Unsupervised” seems to engender the simultaneous wonder, confusion, apathy, and derision that surrounds AI art and generative models. Art critics have referred to “Unsupervised” as a “lava lamp” or “screensaver.” When I talk to people who are artists, scholars, and engineers about AI art, they tend to reflect a dallying attitude—it’s cool, but not earth-shattering, at least not yet. As an art historian by training and ML practitioner by trade, I have mixed feelings.
Some rhetoric around AI art has a techno-optimistic tone: that generative art can drive artists’ creative practices; it can expand imaginative capabilities; it serves as a form of distributive justice. Artwork generated by AI is winning artists’ contests around the world, suggesting it meets standards of taste, and often passing as human-made outputs from other mediums. The frictionless experience of generative tools allows users to create images at greater scale than any human hand could, and they’re only getting better. Midjourney founder David Holz encapsulated the rapid pace of evolution with the phrase “aesthetic accelerationism,” suggesting an influx of new aesthetics due to the infinite permutations of style combinations.
Discussions about the ethics of AI art lately tend to revolve around creativity, labor, privacy, and ownership. These are important and complex ways of framing the generative AI debate, but I think there are more existential concerns. What does the explosion of AI art mean for visual culture? How will generative tools such as DALL-E 2, Stable Diffusion, Midjourney, and Imagen affect how we engage with art? These might seem like outsized questions when most people are using AI for slide decks or art therapy. But we live in a society of the spectacle, where visual language dominates communication, with long-studied connections to issues of power and truth. Generative art models, being dream-machines that can materialize any wish, may reveal a shift in cultural values. When suddenly we have the power to ask for anything, what do we choose?
Many have already pointed out how AI images reflect a creation bias that indicates what’s popular among users, such as requests for specific aesthetics or artists' styles, as well as a curation bias regarding the limited scope of the models’ training data. It’s one thing to say artworks look derivative—Adorno wrote a seminal essay about this experience as a cultural phenomenon in the 1940s—but another to examine how machine learning processes change the very content and quality of images.
Diffusion models abstract their understanding of characteristics and patterns present in training data by correlating visual appearance with descriptive information. These models inherently learn a hierarchy of features to distinguish subjects and styles, such as the presence of waterlilies in a Monet. But in the process, the model tends to devalue what it considers irrelevant or unimportant features, or even ignore them altogether—which feels incongruous with a field notorious for associating minute details with essential meaning.
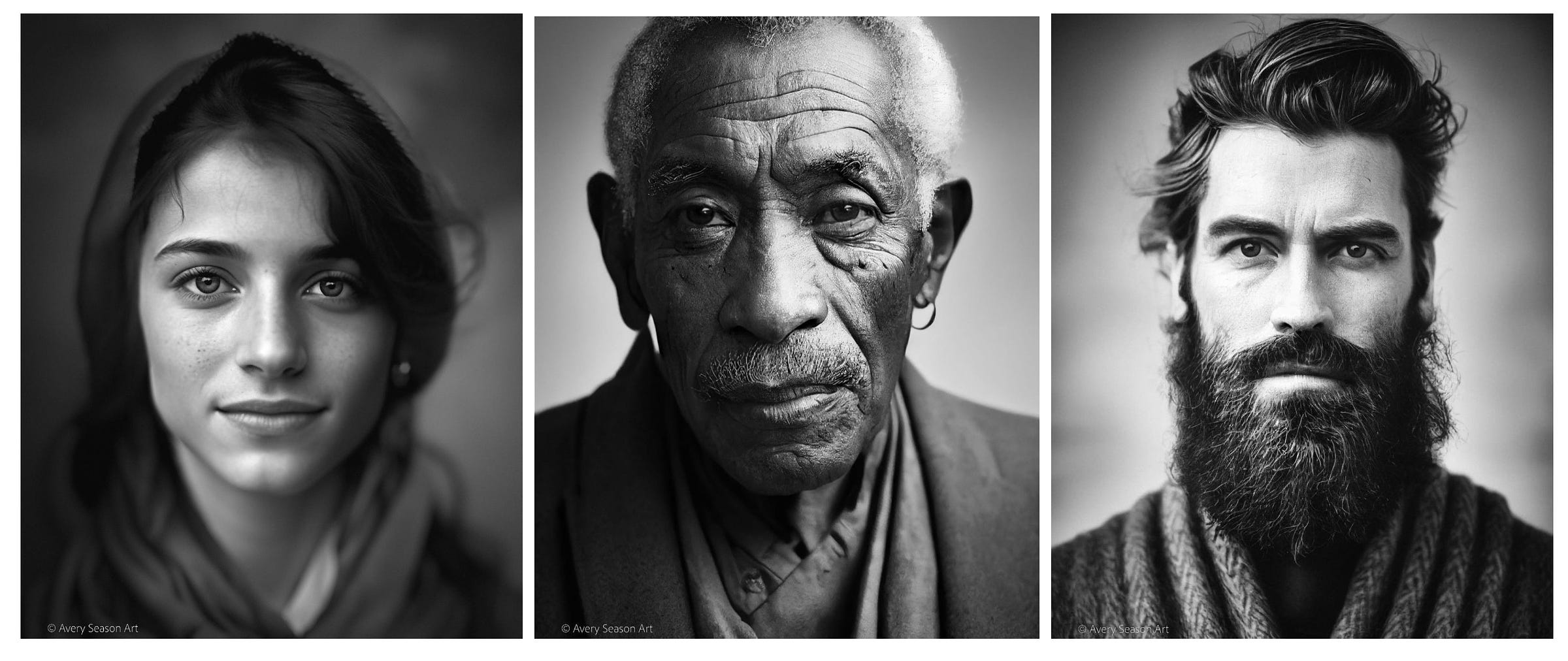
Moreover, the training captions used in these models are scraped from the internet, many of which are prescriptive alt-text that tends to oversimplify, misassociate, and contain biases. They are unlikely to contain rich visual descriptive language to capture artworks’ complexity. The dependence on these captions to comprehend (or compute) a style—the melancholic introspection of a Hopper painting—also leaves little room for diverse subjective experiences and interpretations. The model consequently internalizes implicit concepts such as “beauty” from the dataset. We saw evidence of this in the Lensa avatar trend that lit up social media, where portraits were unintentionally “Yassified,” white-washed, or sexualized. More recently, a popular Instagram photographer confessed that his portraits of symmetrical-faced, well-groomed individuals were AI-generated. These biases and mathematical tradeoffs (such as averaging out representation in a dataset’s samples, or even grading models using—you guessed it—another AI model that predicts humans’ aesthetic judgements) perpetuate narrow ideas of “standard” or homogenous beauty.
Ultimately, models’ assessments of images are merely statistical approximations of the true semantic relationships between image-text relationships. These calculations can fail to capture fine-grained distinctions, leading to blurriness or lack of detail in new images, but on a deeper level, they can inadvertently reduce an artist’s oeuvre to an implicit average unit. The thought is troubling. If we continue to extract the conceptual midpoint of a style, artist’s portfolio, or subject in a latent space, we raise debates about aesthetic standards and risk generating more “average,” or mediocre, images. Even if model design improvements are en route, the process of subjecting art to an algorithm spurs questions about what a standardized representation of art history would entail, and whether one would be desirable.
For David Holz, the aim of a generative art model like Midjourney is clear: “There's no intention in the machine. And our intention has nothing to do with art. We want the world to be more imaginative and we would rather make beautiful things than ugly things.” On one hand, a world filled with only beautiful images sounds rather boring. But regardless of whether Holz thinks Midjourney outputs are art, his parochial perspective underestimates how images, like algorithms, are mediums of influence.
Conceptual artists undermined traditional art engagement by using immaterial forms such as language, instructions, or ephemeral experiences. It was less about what laid bare on the canvas’s surface and more about the idea itself. As though a harbinger of model training practices, in 1974, artist Martha Rosler reflected on the ways captions can mediate biased readings of ambiguous social scenarios in photographs. This shift demanded viewers to rely on not only an aesthetic judgment but also on politically and socially aware ones. In the fast-paced world of AI image reproduction, thinking beyond beauty and pleasure can help us remain attuned to the ways images can be vehicles for critical engagement.
If we can transfer van Gogh’s swirling brushstrokes onto a cat or a cowboy, does the fact that he once chose to paint the starry sky matter less to us? When facing questions about what we can learn or lose from enmeshing motifs and styles, I can’t help but think of the concept of “context collapse.” With regards to AI art, I propose what one might instead think of as “aesthetic collapse,” which consists of merging once distinct styles, historical contexts, and subjects into a souped-up aggregate that challenges our ability to interpret and understand art.
As AI-generated images around us become generalized and abstracted, the same effect may apply to our readings of them. Travis Diehl’s critique of Anadol’s “Unsupervised” is notable: “‘You’d never know that Faith Ringgold’s ‘American People Series #20: Die,’ a bloody tableau of a 1960s uprising, may have provided shades of yellow, brown and red.” For Ringgold, the conditions of that splinter in time, in the midst of the Civil Rights Movement, were inextricable from her practice: “I became fascinated with the ability of art to document the time, place, and cultural identity of the artist. How could I, as an African American woman artist, document what was happening around me?”
When I ask DALL-E 2 for the nonexistent “American People Series #21,” it produces an image of four monumental African American figures. They are dressed in vaguely multicultural clothing, concentrated in prayer while children and community dot the space beneath them. The effect isn’t far from the spurious atmosphere of Disney’s “It’s a Small World”: it suggests harmony, but masks the realities of political and cultural difference. When prompts focus on generating an artistic style, the original reference can lose the unique framework and emotional charge that compelled it into existence. Alternatively, AI art can bury destructive symbolism beneath aesthetic thrill. It might explain why what feels like arbitrary information loss in works like “Unsupervised” tend to leave me with the ghostly feeling that something is missing, or that something is there and I just can’t see it.
Artists have been incorporating machine learning and AI into their artistic practices to unlock socially meaningful insights long before generative models became popular. Hito Steyerl, Anna Ridler, and Trevor Paglen have made prominent work examining our relationships with technology. Adam Chin, a former computer scientist, draws from photographic archives like mugshot databases and vintage photobooth pictures, reimagining them from new angles or in alternative scenarios. His work engages debates surrounding the role of photography, and by extension machine learning, in perpetuating racist stereotypes of criminals (“Front and Profile”) or imagining latent desires across marginalized identities constrained by social pressures (“Photobooth Kiss”).
Chin’s photographs possess a nostalgic and uncanny quality. They offer a glimpse into what the model sees as it traverses a dataset, where the ambiguous shape of a face or body exposes a logical spottiness in determining what should be there compared to the original photograph. Viewers reconstruct the visual narrative of a “criminal”—a blank stare here, a furrowed eyebrow there—but without certainty as to whether their interpretations stem from personal bias or the machine’s algorithm. “Front and Profile” hints at the real-world dangers of “averaging out” faces, especially in non-trivial applications such as policing. Artists like Chin can shed light on the dangers of oversimplifying categories and how machine-generated representations are shaped by, and in turn shape, our perceptions.
Although AI art has existed for decades, our current moment feels especially conducive to the enchantment around generative models. We find ourselves in a world still recovering from the dulling effects of a pandemic, where arts programs are starved of funding, and the line between art and “content” continues to blur. With generative art, you don’t feel like you need to make a “point” so long as it resonates with you. Why should we resist saturating our world with images that mirror the very fabric of our dreams? But if we rely on the machine to dream up, interpret, and remember art, we may be swapping immediate appeal for slower observation, novelty for authenticity, and personalization for social cohesion.
Perhaps AI can equip artists with a bird’s-eye view of society through its ability to parse more cultural data than humanly possible. It can align with what art has always done for humanity—to memorialize, negotiate definitions, and imagine new ways of seeing—as long as we actively shape its meaningful production, creation, and consumption. A start may involve engaging in interdisciplinary conversations on how to quantify the relationships between art and its discourse, as well as larger questions on what we want art to stand for. As AI images continue to paper our world at scale, we have a lot to gain from determining how to sift through meanings clamoring for our attention.
Amanda Wong (they/them) is an AI/ML practitioner, essayist, and art historian based in Oakland, CA. Find them on their website or Instagram.
Acknowledgments: Very grateful to Sarah Miller, Nikhil Devraj, Jessica Dai, Katie Shia, and Wendy Lee for the conversations that helped this piece come together.
Reboot publishes essays and interviews reimagining tech’s future every week. If you liked this and want more, subscribe below.
🌀 microdoses
- ’s Substack has been a masterclass in looking at art. His latest essay explores the value of cultivating ambivalence via composer Julius Eastman and painter Cecily Brown.
Jessica shared this scrollytelling piece about the paintings of Impressionist Berthe Morisot and the gendered context she operated within.
Pitchfork recs but (a little) less pretentious: 10,000 gecs is suggested if you like “Throwing a “gm” in the Discord chat with fingers that smell like gas station corn chips”; the new Caroline Polachek if you’re into “the collected works of theorist Lauren Berlant.”
- released a new fun/depressing web art piece on the tech layoffs.
💝 closing note
Editor applications are due on June 8! This is a part-time, stipended role.
Editors' main responsibility is producing one piece end-to-end every three months, plus supporting and advising each others’ work along the way. We’re looking folks who are clear writers; collaborative thinkers; and deeply, passionately, voraciously curious.
To be an editor is to be a curator and amplifier of ideas that matter. I hope you’ll apply.
Look carefully,
Jasmine & Reboot team